Protein Structure Prediction at CSSB
Proteins are the building blocks of life. Knowledge of a protein’s three-dimensional structure enables scientists to not only determine how the protein functions but also helps reveal how proteins interact with each other and form complex molecular machines.
CSSB scientists investigate complex protein complexes such as Salmonella’s Type III secretion system, the herpesvirus nuclear egress complex or the human nuclear pore complex. Understanding the structure and function of these protein complexes contributes to the identification of new drug targets and the development of novel therapeutics.
This year’s Nobel Prize in Chemistry was recently to David Baker for computational protein design and to Demis Hassabis and John Jumper for protein structure prediction. CSSB scientist use both RoseTTAFold and AlphaFold2, the AI protein structure prediction and design tools created by the Nobel Prize winners, for their own research projects.
In the following interview CSSB scientists Maya Topf (UKE, LIV), Jan Kosinski (EMBL) and Jens Bosse (MHH) share insights about protein structure prediction in their own research …
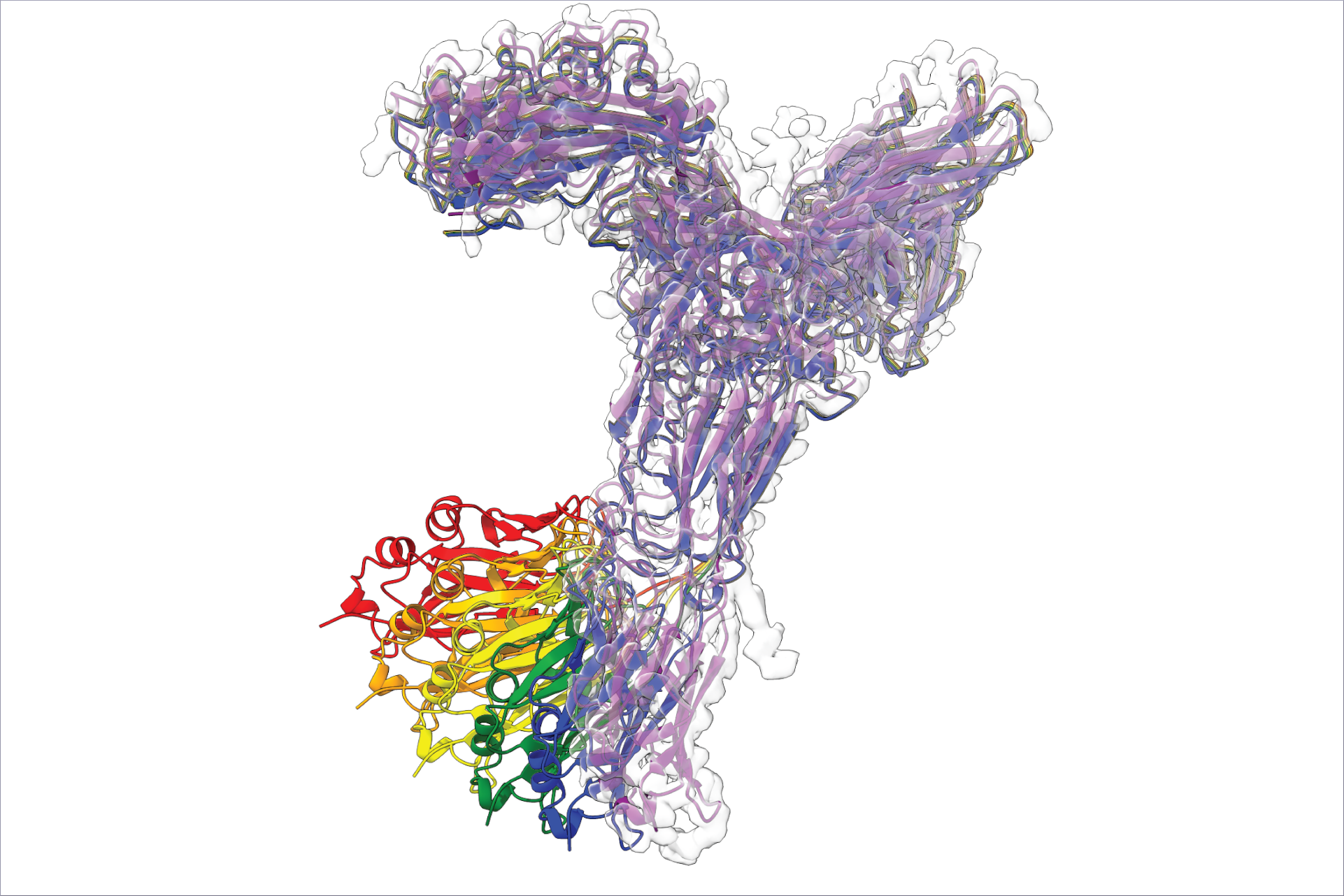
How do you use protein structure prediction in your research at CSSB?
Maya: At the CSSB, we have been using protein structure prediction routinely to interpret cryo electron microscopy (cryoEM) maps, by fitting models into these maps, something we have been doing since the early 2000s. However, since the “AlphaFold” breakthrough in 2020, we can achieve high-accuracy models even for proteins with low or undetectable homology to known structures. As a result, we now apply protein structure prediction more broadly—for instance, to model protein assemblies in HSV1, together with Kay Grünewald and in HCMV, together with Jens Bosse. We also use it in combination with crosslinking mass spectrometry data to calculate ensembles of structures in varying conformations (doi.org/10.1016/j.mcpro.2024.100724). Additionally, we are evaluating the quality of the predicted models using cryoEM data, as part of our contribution to the CASP experiment in structure prediction (doi.org/10.1002/prot.26644).
Jan: In 2021, right after AlphaFold was released, we combined AlphaFold and cryogenic electron tomography to build a new model of the nuclear pore complex, the largest complex in the human cell (https://t1p.de/av5wr). Since then we used it in other projects, for example, in collaboration with Charlotte Utrecht from CSSB and Maria Rosenthal, we mapped how two key proteins of the Lassa virus interact together (https://t1p.de/hgdj5). We’ve also created new tools like AlphaPulldown, which leverages AlphaFold for high-throughput, in-silico protein interaction screens (https://t1p.de/c13om).
Jens: Since the EMBL AlphaFold database is missing viral proteins, we have generated proteome-wide predictions of all human herpesviruses. These predictions are freely available through our Herpesfolds database ( www.HerpesFolds.org/herpesfolds). Moreover, we have used structural similarity searches to cluster the proteins based on their structure into structural groups. Using this approach, we have identified several novel protein families that show conserved and essential functions in human infection (see preprint in BioRxiv)
Utilising AlpahFold Multimer, we have also performed large-scale protein-protein interaction screens of all possible viral protein combinations for four highly important herpesviruses (HSV-1, VZV, HCMV and KSHV, available at www.HerpesFolds.org/herpesppis, about 25000 predictions and 10.000 GPU-hours). Importantly, we find that predicted interactions which are conserved between these viruses are almost perfectly reflecting experimentally known interactions, underlining the predictive power of this appraoch.
How has AlphaFold2 changed the way you conduct your research?
Maya: It increased our confidence in structural predictions significantly, also because the methods provide very reliable scores to tell us what is a good model and what is a bad model. It can also model proteins with little or no homology. This means that we can now explore more complex systems, like multi-protein assemblies, with unprecedented accuracy.
Additionally, the process is a lot faster now, which means that we can explore a lot more viral proteins and protein complexes and potentially identify new drug targets.
Jan: It’s made our work faster and way more exciting.
Jens: AlpahFold has transformed our research significantly as an extremely powerful hypothesis generator that basically is always at the start of a project.
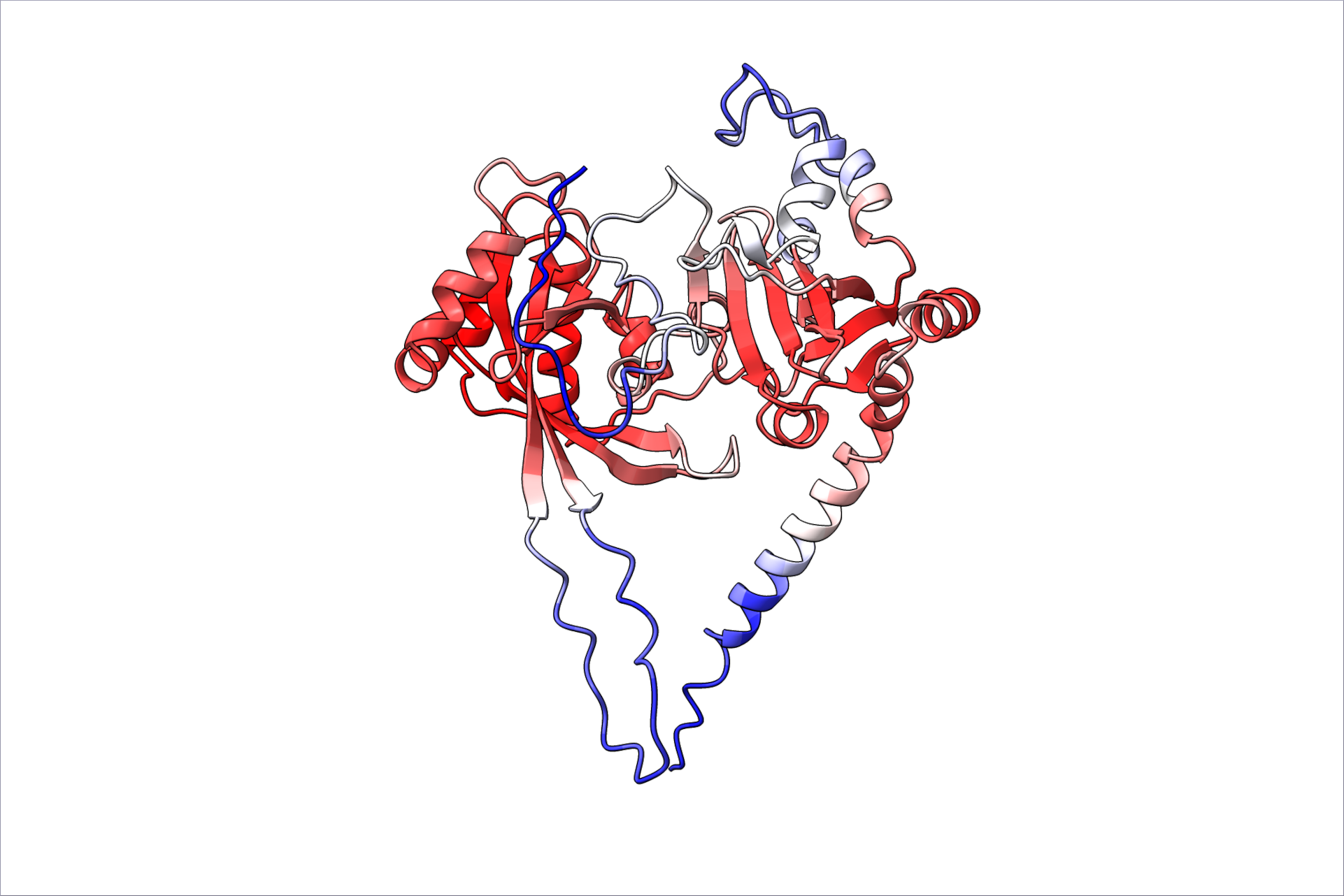
What do expect to be the next breakthroughs in protein structure prediction?
Maya: Maybe the next breakthroughs in protein structure prediction will be in membrane proteins, which remain challenging to predict and are often important drug targets. Additionally, improving predictions of transient and weak protein interactions, including those with small molecules and nucleic acids, will be crucial for better understanding protein function.
Jan: Predicting inter-molecular interactions is still a mix of successes and challenges, but the AI methods for this are rapidly improving. This could be the next big breakthrough.
Jens: While there will be only small further increases in the performance of general protein structure and protein-complex predictions, antibody binding is still a challenge; moreover, interactions with small molecules, DNA and RNA, as well as PTMs, are important challenges. Furthermore, the in-silico design of proteins and binders is still at the beginning, and better algorithms will likely increase the experimental success rate. Finally, the interaction of disordered protein domains is still in its infancy.